On the afternoon of September 23, 2023, the "Fourth Young Scholars Joint Forum" and the "Second Joint Workshop on Big Data and Artificial Intelligence Research Hotspots" were successfully conducted as integral components of the 50th-anniversary lecture series celebrating the discipline development of the School of Computer Science and Technology at Huazhong University of Science and Technology (HUST). The event was jointly organized by the School of Computer Science and Technology at HUST, the School of Computing at the National University of Singapore (NUS), and other world-renowned universities. The event took place in the form of an online Zoom conference.
During the opening ceremony, Professor Xuanhua Shi, Vice Dean of the School of Computer Science and Technology, warmly welcomed the young scholars and top talents participating in the forum. Commemorating the 50th anniversary of the discipline development in computer science and technology, the Young Scholars Joint Forum sought to advance the progress of the discipline, foster diverse collaborations among leading universities, and broaden the global outlook of faculty and students across the university.
Professor Xuanhua Shi provided an overview of the School, encompassing the evolution and development of its disciplinary framework, research platforms, salary and benefits, source of student admissions, and talent cultivation. Additionally, Professor Shi highlighted the favorable working environment within the School and expressed his aspiration for increased collaboration and exchange among all participants
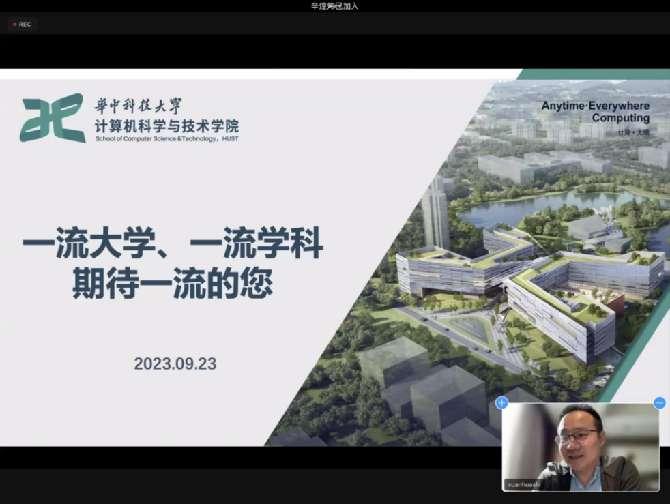
Afterward, a group photo was taken to commemorate the occasion. Everyone expressed their hopes for the complete success of the Fourth Young Scholars Joint Forum and the Second Joint Workshop on Big Data and Artificial Intelligence Research Hotspots, which marked the 50th-anniversary lecture series on the School of Computer Science and Technology discipline development at HUST. The 16 participating guests were divided into four groups following the photo session to engage in academic presentations and discussions.
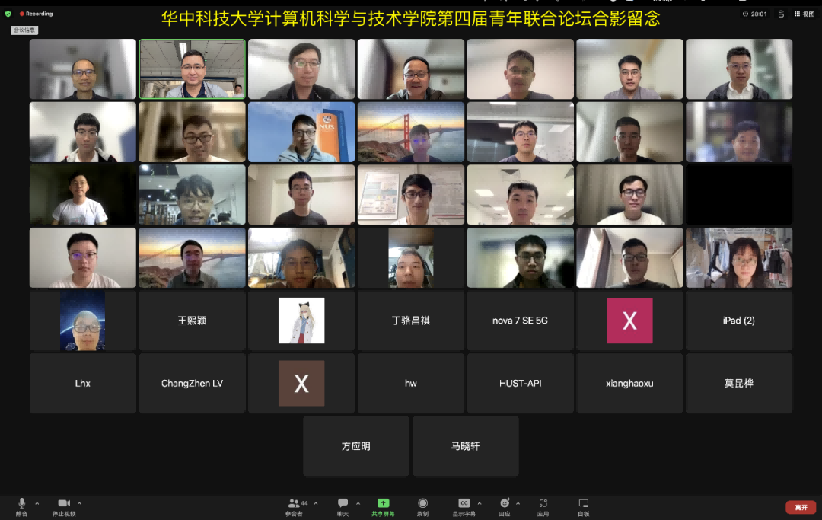
Four presentations were made in the first reporting gourp:
Professor Bingsheng He, Vice Dean of the School of Computing at NUS, started by introducing the original intention behind establishing the Young Scholars Joint Forum, emphasizing the importance of strengthening communication and cooperation among participants and expressing his best wishes to all attendees. He then gave a wonderful and inspiring report on the theme of big data and AI development in the next decade, in which he raised questions about how the industry will develop in the next ten years and gave a short but profound discussion on the history of the development of big data and AI industry, the current problems, the cross-fertilization of big data and AI with other directions, the direction of the future development of the technology as well as the impact of technological advances on the economy and the society. The discussion guided and inspired everyone to think and discuss more.
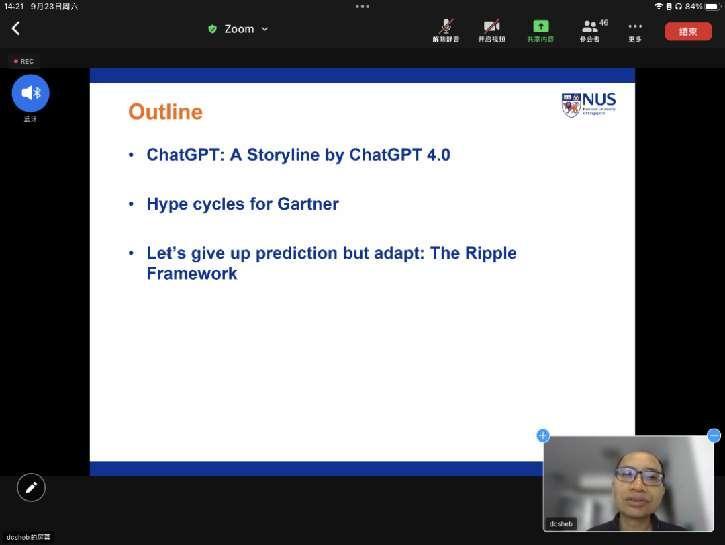
Dr. Zhang Zhen, a postdoctoral researcher at NUS, delivered a report titled "Graph Domain Adaptation: Methods and Applications." The report introduced methods and applications for transferring labeled graph data to unlabeled graph neural networks, such as cross-platform data analysis and cross-graph domain prediction. The proposed approach reduced model training costs and improved the model's generality.
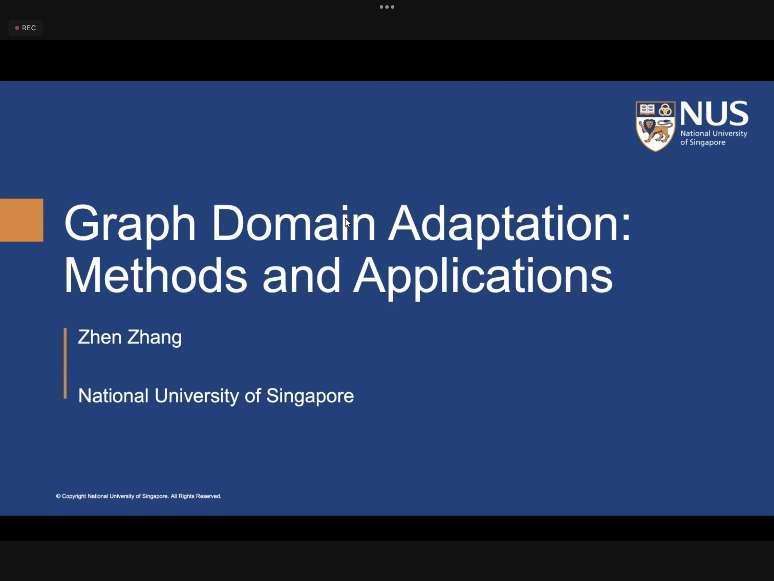
Dr. Zemin Liu, a postdoctoral researcher in the School of Computing at NUS, presented a report titled "Imbalanced Learning on Graphs: Problems, Techniques, and Future Directions." The report discussed the phenomenon of information imbalance and introduced imbalanced learning on graphs in that context. It provided two classification frameworks for the literature on imbalanced learning on graphs: (1) problem classification, which described the forms of imbalance, related tasks, and potential solutions, and (2) technique classification, which detailed critical strategies for addressing these imbalances. The report also discussed future directions for imbalanced learning on graphs.
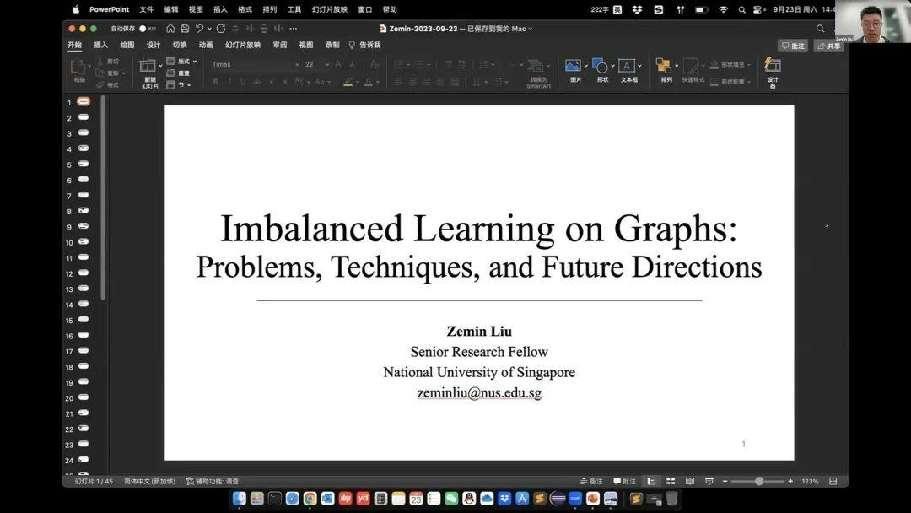
Dr.Jiale Chen, a doctoral student from Nanyang Technological University(NTU), delivered a report titled "Concurrent Artificial Intelligence of Things and Its Industrial Applications." In his presentation, he introduced two methods for improving the efficiency and accuracy of AI systems in the Internet of Things (IoT) through collaborative cooperation among different devices.
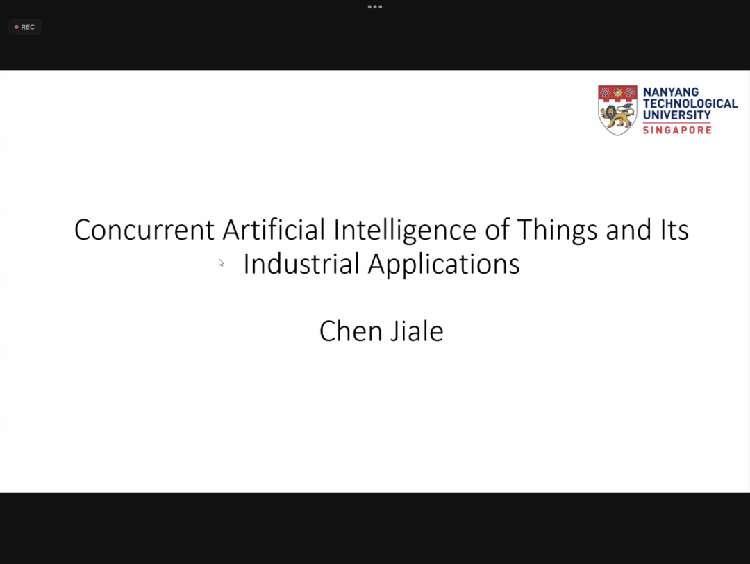
A 10-minute panel discussion was held after completing the first set of reports. The four speakers and the moderator, Professor Yuchong Hu, exchanged and debated the use of graph data in the context of IoT, the requirements of graph neural networks on underlying architectures, and facilitated communication between the graph field and the architectural field. Professor Bingsheng He supplemented his presentation and provided advice to young researchers, encouraging them to focus on in-depth research in a specific area.
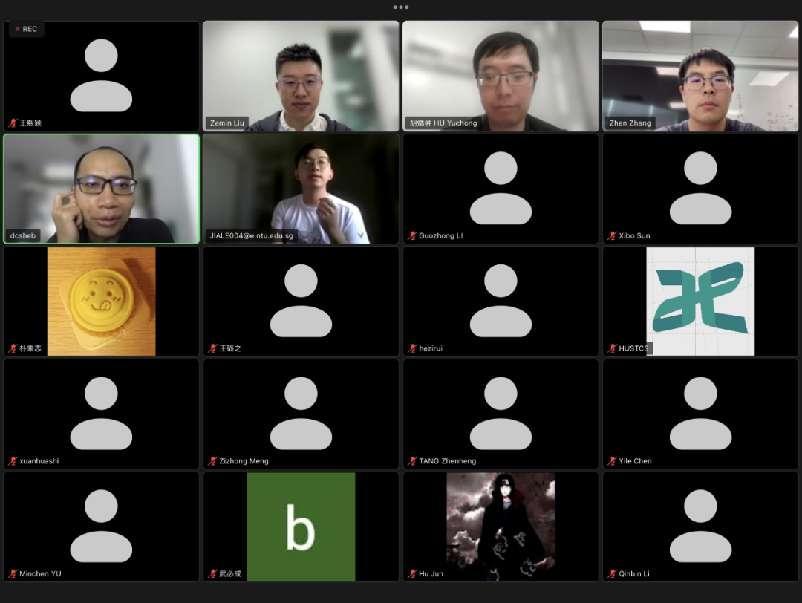
Next was the second group's presentations.
Dr. Yile Chen, a postdoctoral researcher from NTU, delivered a report titled "Towards Better Urban Intelligence: Data Analysis and Mining of Geospatial Entities." In his presentation, he introduced the research background and practical downstream applications, such as mobility recovery and prediction and spatial relationship inference for data mining and analysis on geospatial entity data, such as points of interest and road networks. He concluded by discussing future trends in this field.
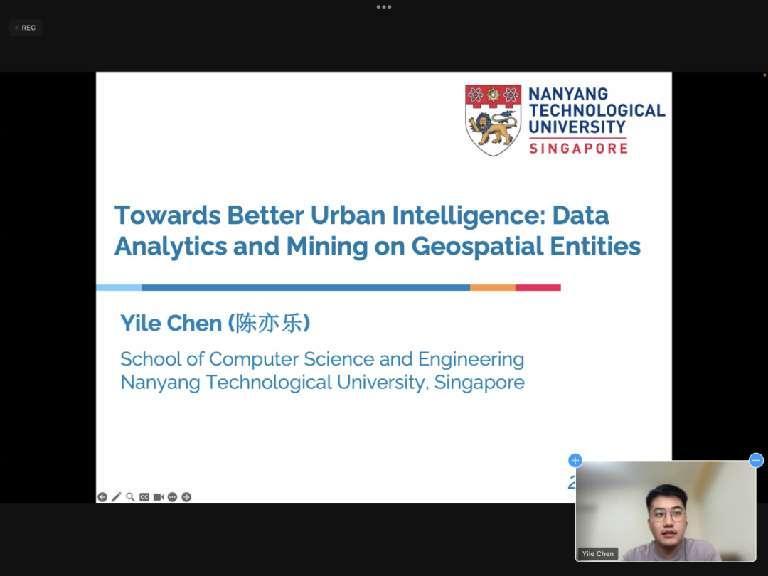
Dr. Kaiqiang Yu, a Ph.D. from the School of Computer Science and Engineering at NTU, presented "Cohesive Subgraph Mining on Large Graphs." His report highlighted the application scenarios of mining cohesive subgraphs on large graphs, such as community search, product recommendation, and fraud detection. He then introduced data mining methods and strategies under two graph structures. His research achievements improved the efficiency and effectiveness of solving similar problems.
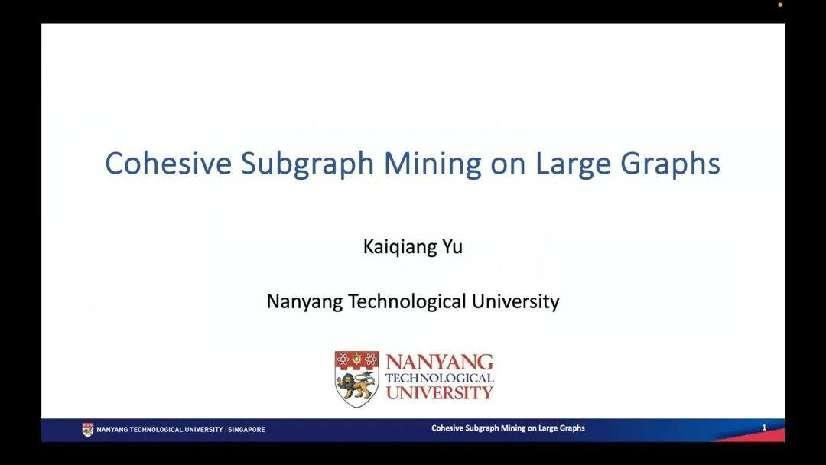
Dr. Jun Hu, a researcher from the School of Computing at NUS, delivered a report titled "Large-scale Heterogeneous Network Representation Learning." In his presentation, he introduced the research background of representation learning for large-scale heterogeneous graph neural networks. He discussed two deep learning methods currently used in this field, along with their respective advantages and disadvantages. His research achievements combined these two methods, resulting in low information loss and highly efficient and effective outcomes.
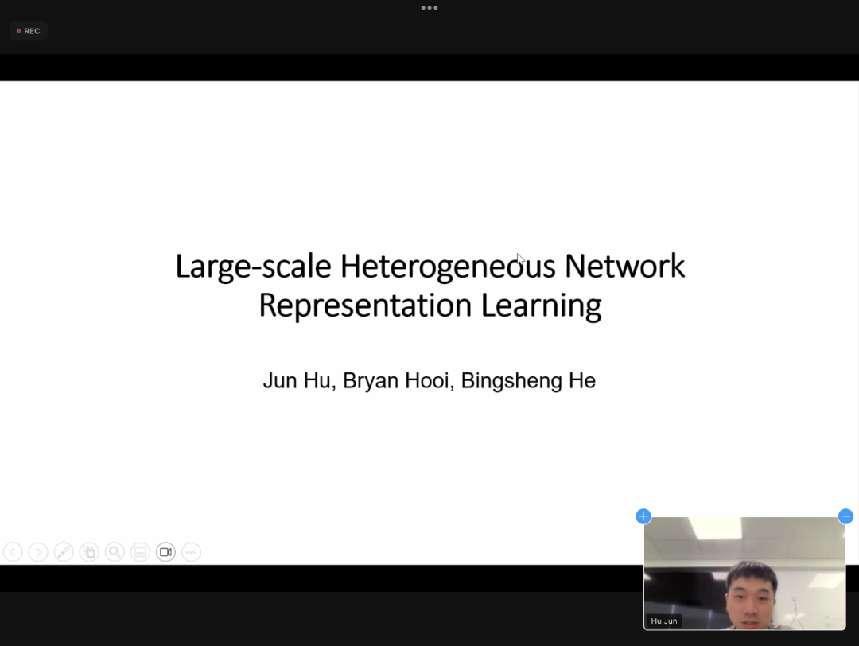
Dr. Jiaxin Jiang, a researcher from the School of Computing at NUS, presented a report titled "Efficient and Effective Graph Algorithms for Fraud Detection." In his presentation, he introduced the graph algorithm called Spade for fraud detection in transaction networks. Fraud detection faces challenges in terms of real-time processing and algorithm complexity. In this context, his research optimized the existing algorithms, achieving improved speed and enhanced effectiveness in dynamic graph scenarios, as well as exponential enhancement in static graph scenarios. Lastly, he introduced other research achievements of his team and invited everyone to strengthen communication and collaboration.
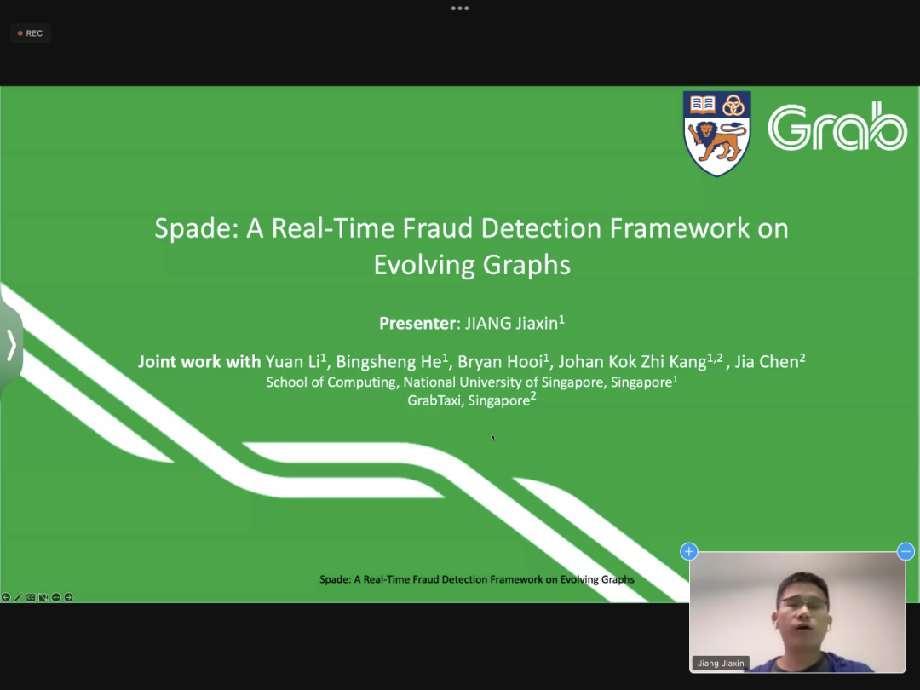
Then, the four speakers of the second group and the moderator Professor Yuchong Hu, had a panel discussion on the content of the second group's report. The discussion covered topics such as subgraph detection and road networks in the temporal dimension, resulting in positive outcomes and fruitful discussions.
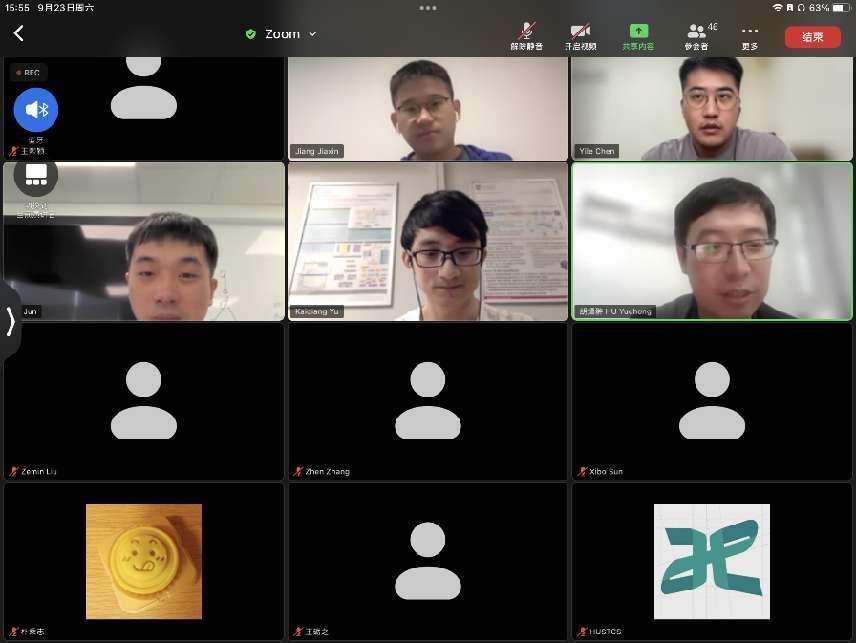
The third group of reports follows:
Dr. Keke Huang, a researcher in the School of Computing at NUS, delivered a presentation entitled "Node-wise Diffusion for Scalable Graph Learning." He introduced a node-based diffusion algorithm for large-scale graph learning in his report. Specifically, he discussed a general node diffusion model (NDM) and a scalable graph neural network (NIGCN) for node classification. The research results showed excellent performance, particularly on large graphs. Notably, NIGCN achieved representation generation and training completion within 10 seconds on datasets with hundreds of millions of nodes and billions of edges.
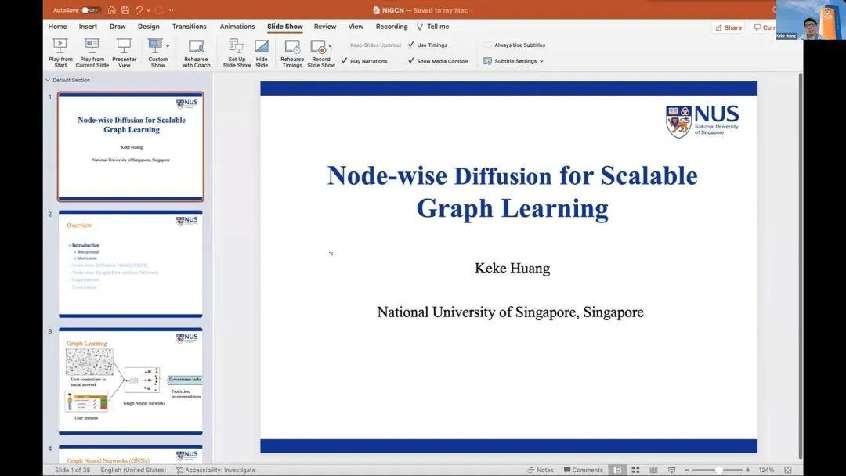
Dr. Qiange Wang, a postdoctoral researcher at NUS, presented on "Scalable GPU-accelerated graph neural network training method." His report introduced a GPU-accelerated solution for large-scale graph training. The solution utilized a hybrid training framework of CPU data offloading and recomputation caching, along with data access optimization based on communication redundant data elimination. With a limited number of GPUs, the method efficiently executed full-batch training on graphs with billions of vertices. The research achievement reduced the overhead of intermediate results and decreased communication costs. It demonstrated a significant speedup compared to previous algorithms, yielding promising results.
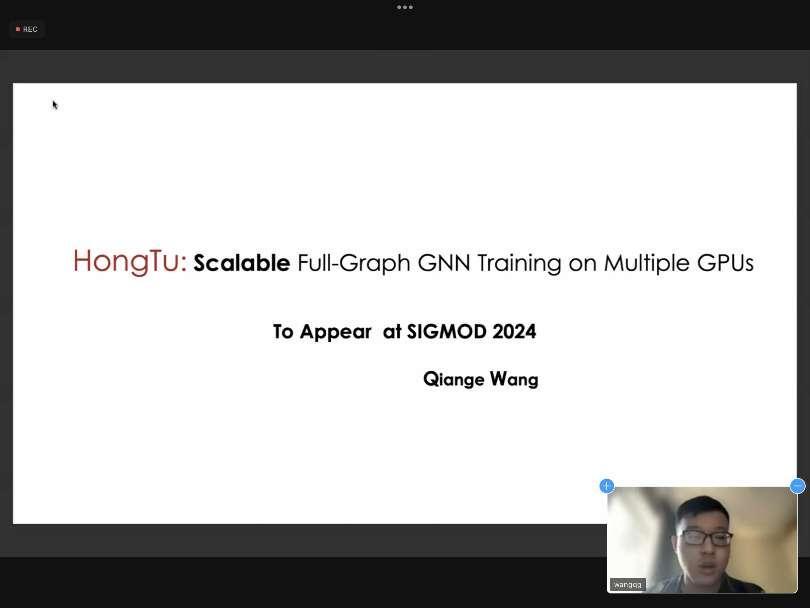
Dr. Zizhong Meng, a Ph.D. in the School of Computer Science and Engineering at Nanyang Technological University (NTU), Singapore, presented "Enhancing base estimation performance using machine learning techniques." His report provided an overview of the research background, challenges, and proposed solutions. The research results reduced the domain size during learning, significantly improving learning accuracy and reducing learning time. Finally, he discussed future directions for his research.
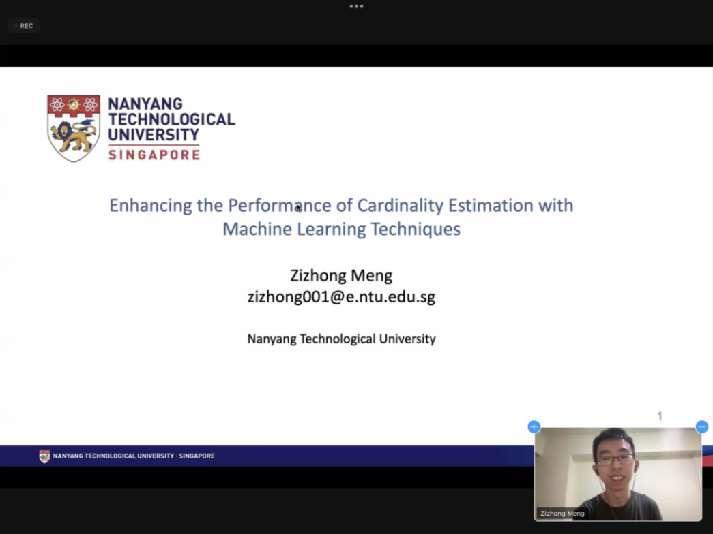
Dr. Guozhong Li, a Postdoctoral Fellow in the Computer Science Department at King Abdullah University of Science and Technology (KAUST) in Saudi Arabia, delivered a presentation titled "ShapeNet: A Shapelet-Neural Network Approach for Multivariate Time Series Classification." In his report, he discussed the research background, challenges, current progress, and prospects of ShapeNet.
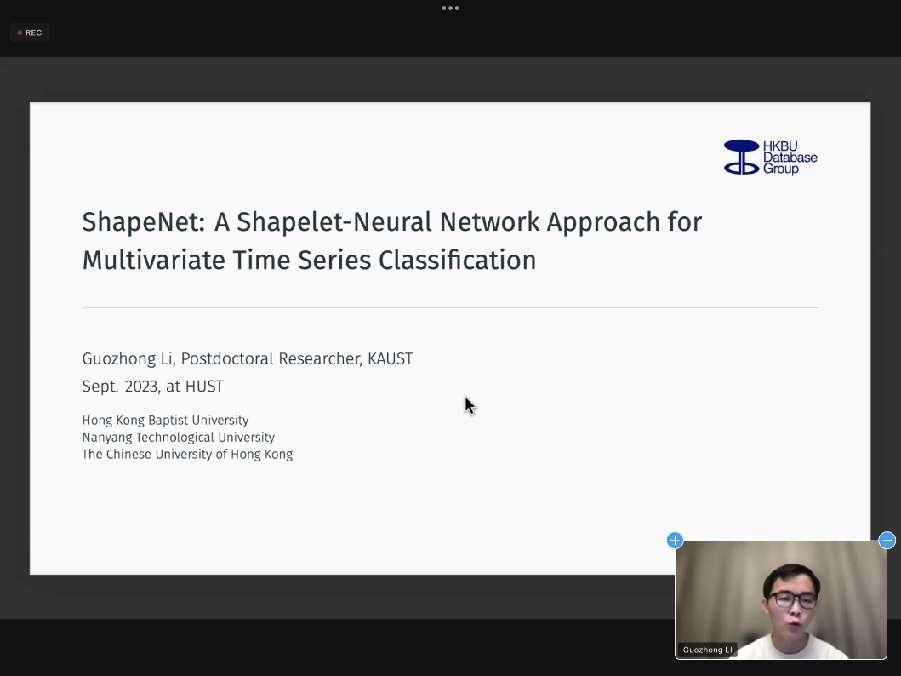
Following the presentations, the four speakers and Professor Yuchong Hu, the moderator of group reporting, engaged in a panel discussion on the topics raised in the third group's reports. The discussion covered the advantages of graph neural networks compared to traditional ones, partition issues in graph training, and timing concerns regarding CPU uploading checkpoints, yielding positive outcomes.
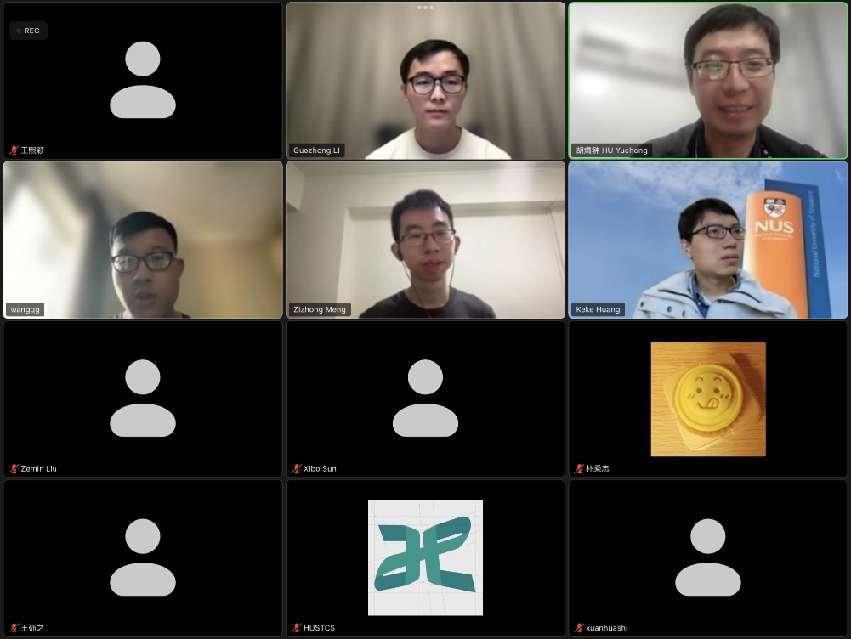
Next was the fourth group of presentations.
Dr. Chengzhi Piao, a postdoctoral researcher at The Chinese University of Hong Kong(CUHK), delivered a report titled "Computing Graph Edit Distance via Neural Graph Matching." In his statement, he proposed a new model framework that transforms the original graph into the target graph in as few steps as possible. This achievement provides an inexact solution for calculating the distance in graph editing.
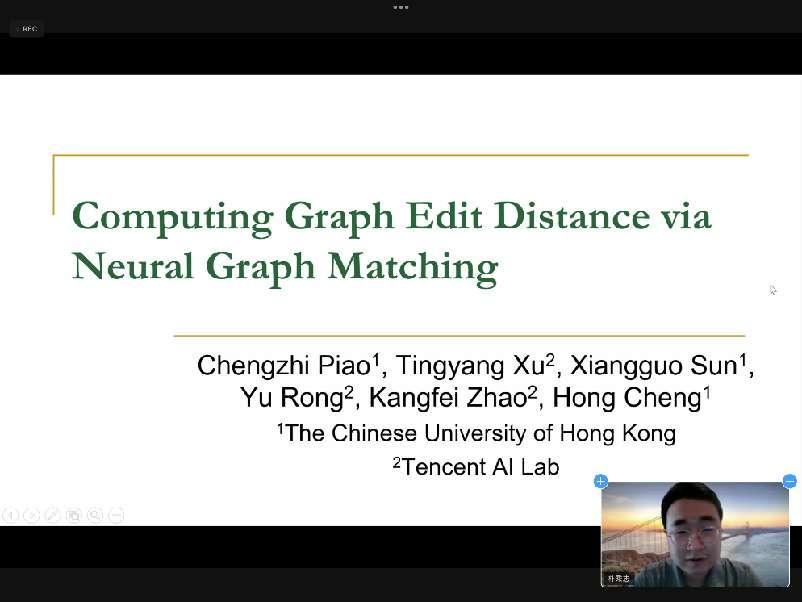
Dr. Xibo Sun, a PhD in the Department of Computer Science and Engineering at Hong Kong University of Science and Technology (HKUST), presented a report entitled "Efficient GPU-Accelerated Subgraph Matching." He introduced the latest research findings from his team, offering an algorithm called EGSM for subgraph matching based on GPU. Furthermore, they employed a parallelized hybrid BFS-DFS strategy, resulting in faster execution and broader problem-solving capabilities. This algorithm significantly outperforms state-of-the-art GPU-accelerated algorithms.
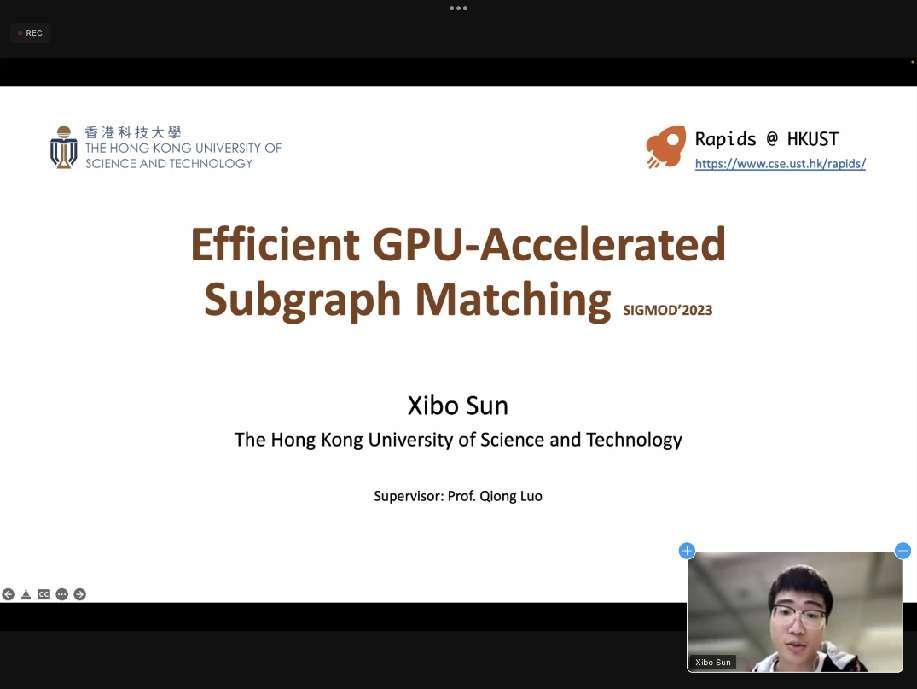
Dr. Zhenheng Tang, a PhD at Hong Kong Baptist University (HKBU), delivered a report titled "Efficient and Effective Federated Learning under Data and System Heterogeneity." In his report, he discussed the background of federated learning and its current challenges. To address data heterogeneity, his team proposed virtual homogeneous learning, which uses a common noisy dataset to correct the data feature representations of different models. This approach dramatically improves training convergence speed and generalization ability. He proposed a bandwidth-based joint learning communication scheduling algorithm for communication issues in system heterogeneity, reducing communication time.
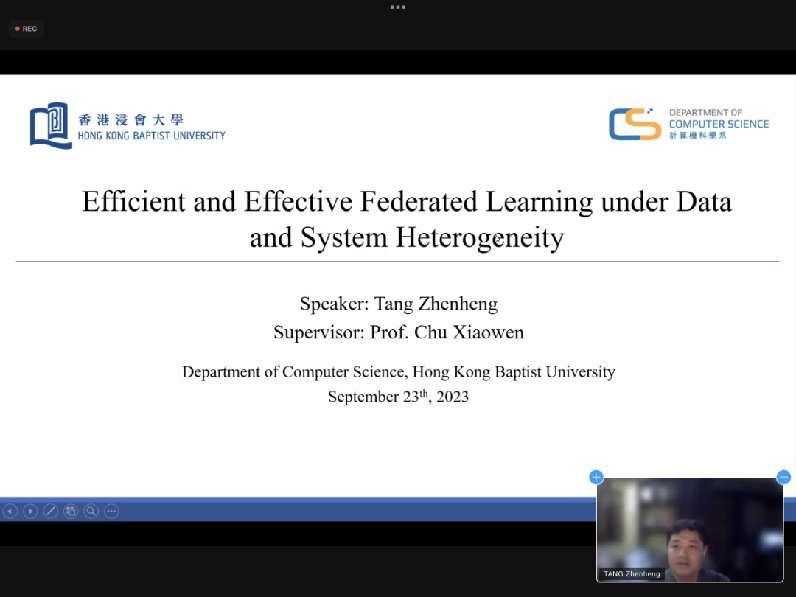
Dr. Minchen Yu, a researcher who obtained a doctoral degree from HKUST, presented a report titled "Following the Data, Not the Function: Rethinking Function Orchestration in Serverless Computing." In his statement, he introduced the background of serverless computing. He pointed out the lack of expressive power, usability, and practicality in serverless function orchestration platforms. Based on a data-centric function orchestration concept, he designed a serverless platform to implement complex applications easily, achieve low-latency function interaction and data exchange, and yield significant improvements.
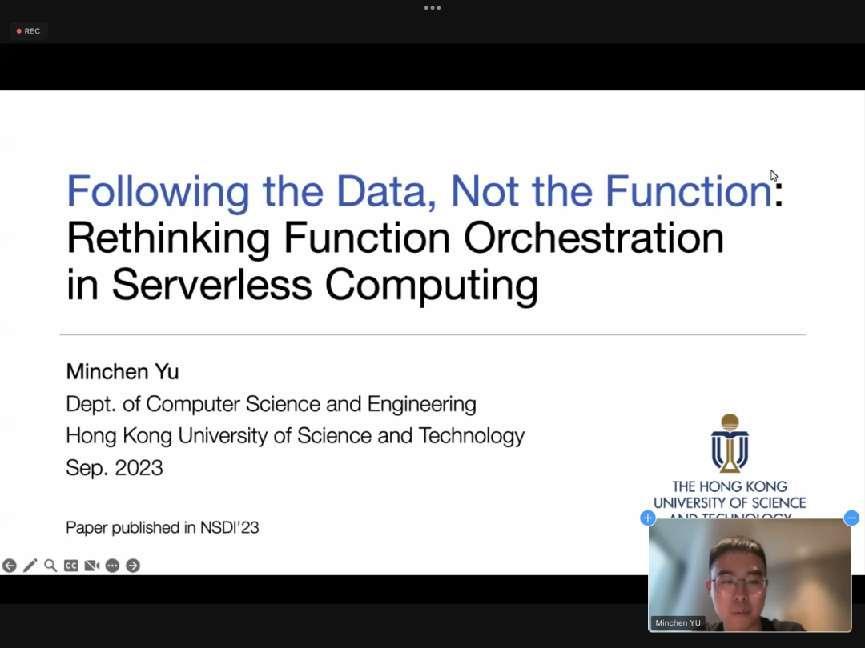
Following the presentations, the four speakers from the fourth group and Professor Yuchong Hu, moderator of group reporting, engaged in a panel discussion. The discussion covered topics such as GPU memory issues in processing, the decreasing solution space, and the increasing time complexity of NP-hard algorithms during practical computations and achieved positive outcomes.
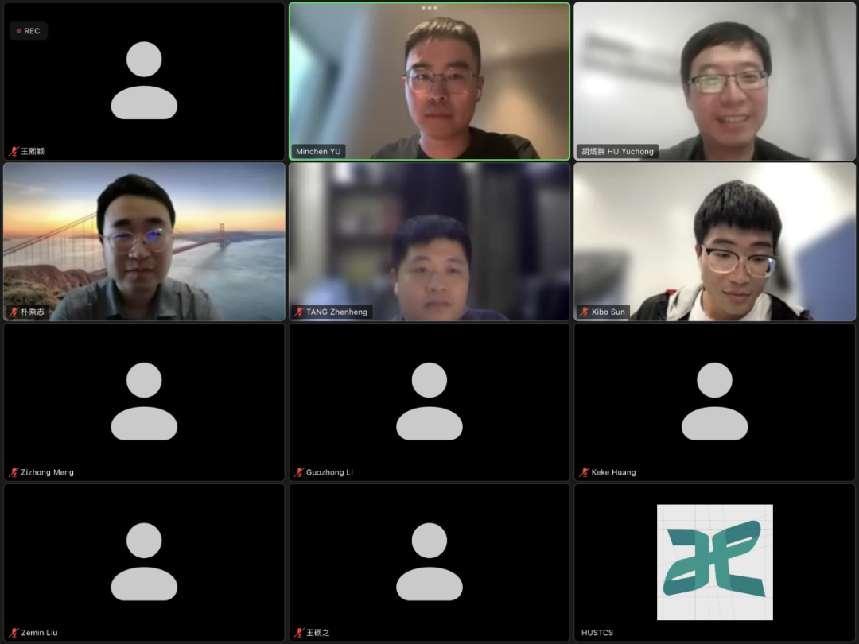
This Workshop focused on hot research topics in graph algorithms, federated learning, serverless computing, and data mining in the context of big data and artificial intelligence. It facilitated academic exchanges among young scholars and students, broadened the international perspectives of our faculty and students, and laid a solid foundation for deep collaboration between the universities.