Recently, two undergraduate students from our university, Chen Dongping, a 2021 Big Data major, and Lu Runyu, a 2020 Excellence Program student, had their papers accepted by the prestigious International Conference on Machine Learning (ICML).
ICML, one of the three top-tier machine learning conferences, received 9,473 submissions this year, with an acceptance rate of only 27.5%. Recognized as an "A" level conference by the China Computer Federation (CCF), ICML is committed to publishing high-quality, innovative research and rapidly disseminating top research achievements within the machine learning community.
Chen Dongping, a 2021 Big Data major, and Liu Yinuo, a 2022 Artificial Intelligence major from the School of Artificial Intelligence and Automation, were the co-first authors of the research paper titled "MLLM-as-a-Judge: Assessing Multimodal LLM-as-a-Judge with Vision-Language Benchmark." Guided by Associate Professor Wan Yao of Huazhong University of Science and Technology, their work distinguished itself in a rigorous review process, leading to an invitation to present at the ICML conference in Vienna in July 2024.
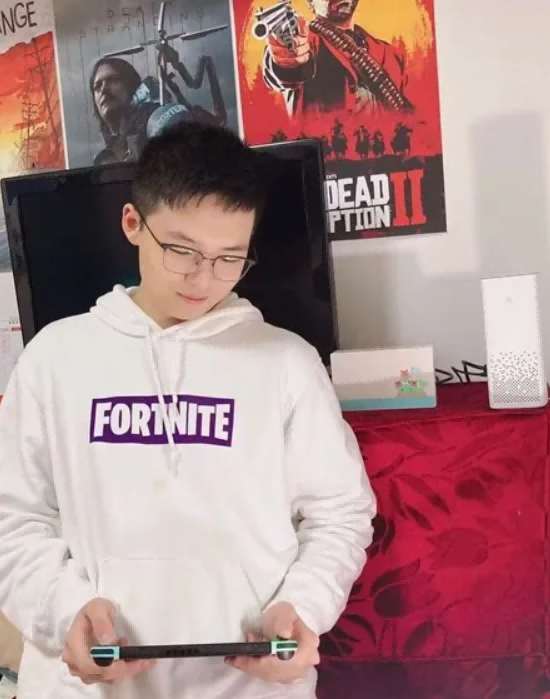
Chen Dongping, a 2021 undergraduate majoring in big data
The HUST research team was the first to propose and develop a new benchmark, MLLM-AS-A-JUDGE, to evaluate multimodal large language models (MLLMs). The research not only focuses on three core tasks;scoring assessment, pairwise comparison, and batch ranking;but also delves into the performance of MLLMs in these judgment tasks. Additionally, this work is the first to thoroughly analyze the use of multimodal large language models as evaluators, addressing issues such as bias and hallucination that may arise during task execution. To gain a more comprehensive understanding of MLLMs' capabilities and limitations, the study also investigates how large language models (LLMs) handle and assess multimodal content without visual input. This aspect of the research enhances our understanding of how models make judgments when specific perceptual inputs are missing, and challenges the effectiveness of current technologies in scenarios that do not solely rely on visual information.
The experimental results revealed several key findings. While MLLMs, such as GPT-4V, exhibit human-like judgment in pairwise comparison tasks, their performance significantly deviates from human preferences in scoring assessment and batch ranking tasks. Moreover, although MLLMs excel at handling multimodal information (e.g. text, images, videos), they are still prone to bias, hallucination responses, and inconsistency during judgment processes.
Two datasets were released to advance further research in this domain: MLLM-AS-A-JUDGE-HQ, offering model responses closely aligning with human judgment, and MLLM-AS-A-JUDGE-HARD, highlighting responses with notable discrepancies. These datasets provide valuable scenarios for future investigations.
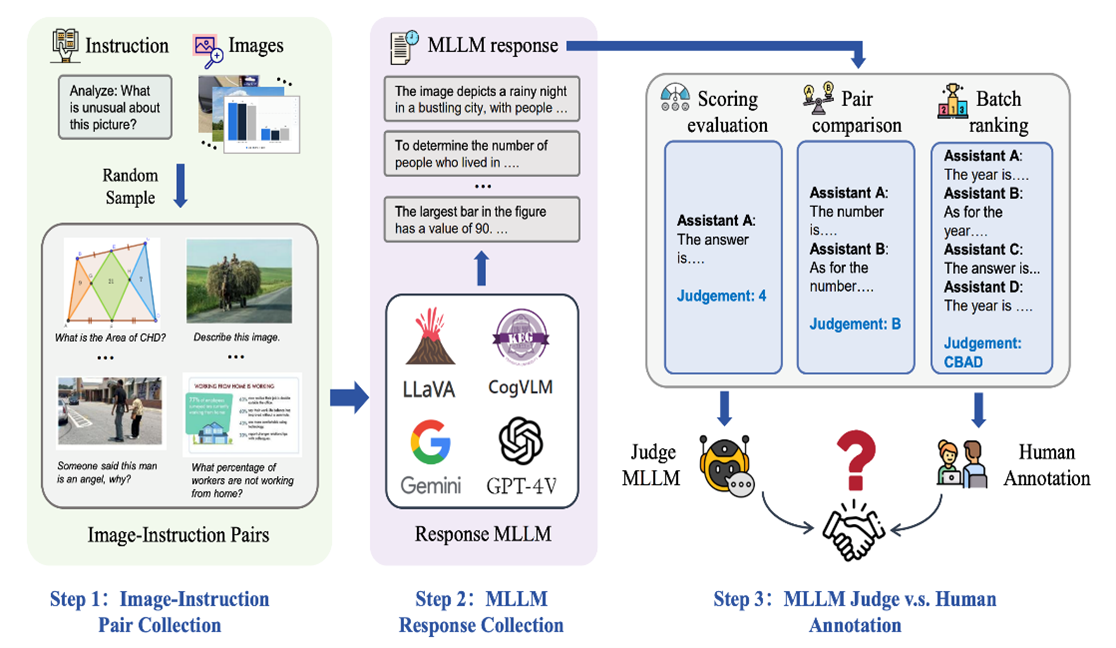
The Research:MLLM-as-a-Judge: Assessing Multimodal LLM-as-a-Judge with Vision-Language Benchmark;
This research was completed under the joint supervision of Associate Professor Wan Yao from Huazhong University of Science and Technology and Professor Sun Lichao from Lehigh University, USA, with support from the National Natural Science Foundation of China. In light of the rapid development of large model research, the team hopes their findings will inspire and contribute valuable insights to the global AI research community.
For more information, visit the research’s official website: https://mllm-judge.github.io/