At the GECCO 2023 International Conference on Evolutionary Computing, team from School of Computer Science & Technology, HUST won the championship in the track of Machine Learning-based Evolutionary Algorithm for Solving Vehicle Routing Problems (ML4VRP).The winning team, comprising Shipei Zhou (graduate student 2022), Chi Zhang (graduate student 2023), Zeyuan Qiu (graduate student 2021) , and Dongping Hou (graduate student 2021) , demonstrated their expertise under the guidance of Associate Professor Yan Jin.
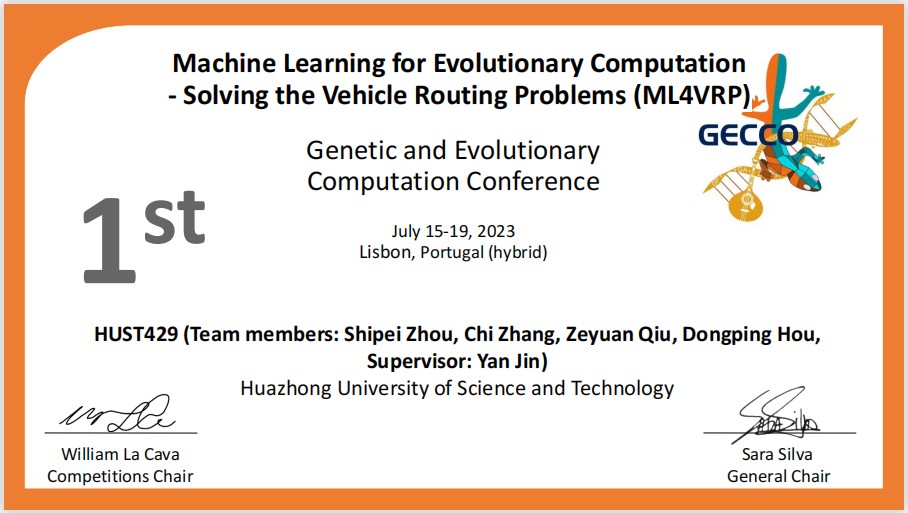
GECCO conference (The Genetic and Evolutionary Computation Conference) is organized by ACM and listed as one of the crucial international academic conferences by CCF. GECCO conference has been one of the annual grand events in the field of evolutionary computation since its establishment in 1999. This year, researchers and top scholars from renowned institutions in countries such as the UK and France congregated to this grand event, demonstrating the latest advancements in evolutionary computation techniques such as genetic algorithms, evolutionary strategies, genetic programming, and differential evolution in various fields.
The Challenge on Machine Learning for Evolutionary Computation-Solving the Vehicle Routing Problems aims to promote the integration of machine learning and evolutionary algorithms in order to effectively address the Vehicle Routing Problems with Time Windows (VRPTW). This problem involves determining the optimal route and driving order for a group of vehicles, with the objective of minimizing total distance or cost, while also satisfying time window and capacity constraints imposed by the customers. The VRPTW problem has practical applications in logistics distribution and cargo collection, offering the potential to greatly enhance transportation efficiency, resource utilization, and customer satisfaction. While this problem has been extensively studied for many years, current research is focused on developing machine learning-based algorithms, which have the potential to overcome the limitations of traditional algorithms and provide innovative solutions.
The winning deep network model, DeepHGS, makes full use of the respective advantages of deep learning and evolutionary computation-solving. By exploiting the "permutation invariance" of the vehicle routing problem, the neural network is effectively integrated with the traditional evolutionary algorithm HGS, achieving significant breakthroughs in the prediction of neighborhood search and high-quality offspring selection, etc. The prediction model can select more promising starting points for local search and accelerate the search process. The order of inputting subpaths does not affect the network's output, enabling the network to learn the characteristics of specific node combinations. The DeepHGS model performs excellently on datasets such as Solomon, Homberger & Gehring, surpassing other competing teams and providing feasible solutions for solving complex real-world problems.